Are you looking for the information related to healthcare sector? Hospaccx healthcare consultancy has mapped all the information related to how Ai is useful in healthcare sector.
This is the superficial and macro level survey if you need a refined market and financial feasibility or any other study related to healthcare is required you can contact Hospaccx Healthcare business consulting Pvt. ltd on Hospaccx.India@gmail.com or you can visit our website on hospaccxconsulting.com
INTRODUCTION:
Artificial intelligence (AI) and related technologies are increasingly prevalent in business and society, and are beginning to be applied to healthcare. These technologies have the potential to transfer many aspects of patient care, as well as administrative process within provider, pair and pharmaceutical organizations.
Considering the advancement in artificial intelligence (AI), Hospaccx Healthcare has explained that how AI proves to be beneficial for healthcare industry.
There are already a number of research studies suggesting that AI can perform as well as or better than humans at key healthcare tasks, such as diagnosing disease. Today, algorithms are already outperforming radiologists at spotting malignant tumors, and guiding researches in how to construct cohorts for costly clinical trials. However, for a variety of reasons, we believe that it will be many years before AI replaces humans for broad medical process domains.
Artificial intelligence is not one technology but rather a collection of them. Most of these technologies have immediate relevance to the healthcare field, but the specific processes and tasks they support vary widely. Some particular AI technologies of high importance to healthcare are defined and describe below.
BRINGING MACHINE LEARNING-NEURAL NETWORKS AND DEEP LEARNING:
Machine learning is a statistical technique for fitting models of data and to ‘learn’ by traing models with data. Machine learning is one of the most common forms of AI. It is a broad technique at the core of many approaches to AI and there are many versions of it.
The most complex forms of machine learning involved deep learning, or neural network models with many levels of features or variables that predict outcomes. There may be thousands of hidden features in such models, which are uncovered by the faster processing of today’s graphics processing units and cloud architectures. A common application of deep learning in healthcare is recognition of potentially cancerous lesions in radiology images. Deep learning is increasingly being applied to radiomics, or the detection of clinically relevant features in imaging data beyond what can be perceived by the human eye. Both radiomics and deep learning are most commonly found in oncology oriented image analysis. Their combination appears to promise greater accuracy in diagnosis than the previous generation of automated tools for image analysis, known as computer-aided detection or CAD.
Deep learning is also increasingly used for speech recognition and, as such, is a form of neutral language processing (NLP), describe below. Unlike earlier forms of statistical analysis, each feature in a deep learning model typically has little meaning to a human observer. As a result, the explanation of the models outcome may be very difficult or impossible to interpret.
THE WORK OF NATURAL LANGUAGE PROCESSING IN AI:
Making sense of human language has been a goal of AI researchers since the 1950s. This field, NLP, includes applications such as speech recognition, test analysis, translation and other goals related to language. There are two basic approaches to it: statistical and semantic NLP. Statistical NLP is based on machine learning (deep learning neural networks in particular) and has contributes to a recent increase in accuracy of recognition. It requires a large “corpus” or body of language from which to learn.
In healthcare, the dominant applications of NLP involved the creation, understanding and classification of clinical documentation and publish research. NLP systems can analyze under structure clinical notes on patients, prepare reports (e.g. on radiology examination), transcript patient instructions and conduct conversational AI.
USE OF PHYSICAL ROBOTS IN HEALTHCARE:
Physical robots are well known by this point, given that more than 2lakhs industrial robots are installed each year around the world. They perform predefined tasks like lifting, repositioning, welding or assembling objects in places like factories and ware houses, and delivering supplies in hospital. More recently, robots have become more collaborative with humans and are more easily trained by moving through a desired task. They are also becoming more intelligent as other AI capabilities are being embedded in their “brains” (operating systems). Overtime, it seems likely that the same improvements in intelligence that we’ve seen in other areas of AI would be incorporated into physical robots.
Surgical robots, essentially approved in the USA 2000, provide ‘super power’ to surgeons, improving their ability to see, create precise and minimally invasive incisions, stitch wounds and so forth. Important decisions are still made by human surgeons, however. Common surgical procedures using robotic surgery include gynecologic surgery, prostrate surgery and head and neck surgery.
MAKING ADMINISTRATIVE APPLICATIONS EASY:
The use of AI is somewhat less potentially revolutionary in this domain as compared to patient care, but it can provide substantial efficiencies. These are needed in healthcare because, for example, the average nurse spends 25% of work time on regulatory and administrative activities. The technology that is most likely to be relevant to this objective is RPA. It can be used for a variety of applications in healthcare, including claims processing, clinical documentation, revenue cycle management and medical record management.
Some healthcare organization has also experimented with chatbots for patient interaction, mental health and wellness and telehealth. These NLP based applications may be useful for simple transactions like refilling prescriptions or making appointments. Another AI technology with relevance to claim and payment administration is machine learning, which can be used for probabilistic matching of data across different data bases.
TELEHEALTH:
The increase of telemedicine has shown the rise of possible AI applications. The ability to monitor patients using AI may allow for the communication of information to physicians if possible disease activity may have occurred. A wearable device may allow for constant monitoring of a patient and also allow for the ability to notice changes that may be less distinguishable by humans.
WHAT IS THE FUTURE OF AI IN HEALTHCARE? :
We believe that AI has an important role to play in the healthcare offering of the future. In the form of machine learning, it is the primary capability behind the development of precision medicine, widely agreed to be a sorely needed advance in care. Although early efforts at providing diagnosis and treatment recommendations have proven challenging, we accept that AI will ultimately master than domain as well. Given the rapid advances in AI for imaging analysis, it seems likely that most radiology and pathology images will be examine at some point by a machine. Speech and text recognition are already employed for task like patient communication and capture for clinical notes and their uses will increase.
The use of AI is predicted to decrease medical costs as there will be more accuracy in diagnosis and better predictions in the treatment plan as well as more prevention of diseases.
CONCLUSION:
Artificial intelligence (AI) is definitely improving the healthcare industry. From predictive medical care and more accurate diagnosis to motivating the patients to take care of their health. AI will certainly continue enhancing the patient experience and healthcare expertise in general.
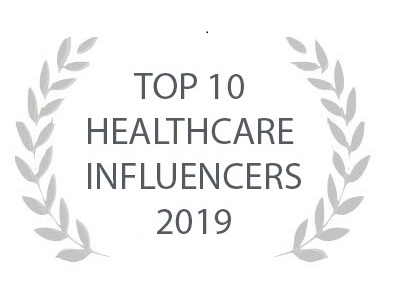
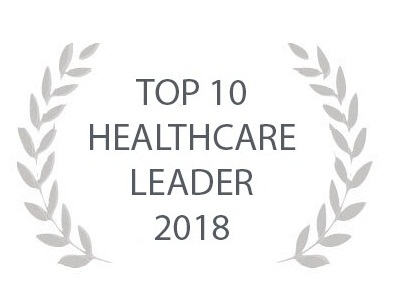
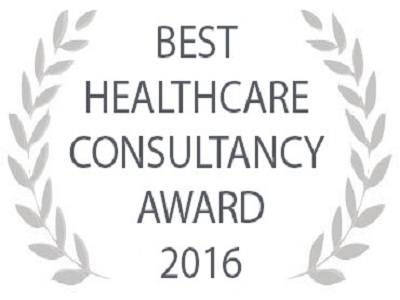
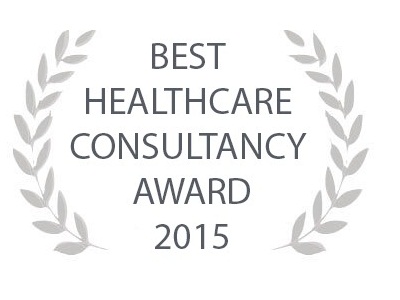
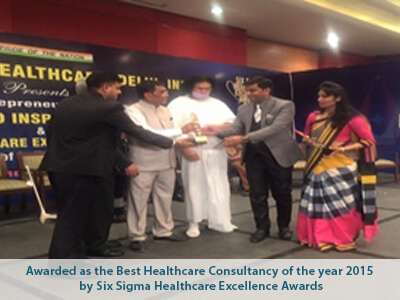
Related Team Members